The Pattern-Seeking Fallacy
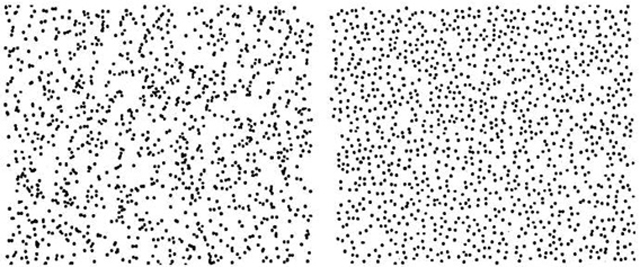
Which of these sets of dots were placed randomly?
We think “right,” but the answer is “left.” Humans are bad at seeing the difference between patterns and randomness.
(From Steven Pinker’s The Better Angels of Our Nature 2011)
What do these have in common?
- “This pitcher has retired 5 of the last 7 batters.”
- “We tried 10 AdWords variants and combination D is the clear winner.”
- “The Bible Code predicted the Sept 11 attacks 5,000 years ago.”
- “We sliced our Google Analytics data every which way, and these 4 patterns emerged.”
All are examples of a common fallacy that I’m dubbing the “Pattern-Seeker.”
You probably laugh at Nostradamus, yet it’s likely you’re committing the same error with your own data.
Patterns in Chaos
It’s commonly said that basketball players are “streaky”—they get on a roll hitting 3-pointers (have a “hot hand”) or develop a funk where they can’t seem to land a shot (“gone cold”). These observations are made by fans, announcers, pundits, and the players themselves.
In 1985 Thomas Gilovich tested whether players really did exhibit streaky behavior. It’s simple—just record hits and misses in strings like: HMHHMMMMHMMHH
, then use standard statistical tests to measure whether those strings are typical of a random process, or whether there is something more systematic going on.
Turns out players are not streaky1; simply flipping a coin produces the same sort of runs of H
’s and M
’s. The scientists gleefully explained this result to basketball pundits; the pundits remained unimpressed and unconvinced. (Surprised?)
1 Editor’s Note: Eight years after this article was published, a critical analysis of the Gilovich data suggested that perhaps there is such a thing as the “hot hand.” Others joined the fray; the final result is inconclusive. More from Wikipedia. What we can conclude, is that it’s extremely difficult to know whether something is random, even when you’re a paper-writing statistician.
So they tried the same experiment backward: They created their own strings of H
’s and M
’s with varying degrees of true streakiness and showed those to pundits and fans, asking them to classify which were streaky and which were random. Again they failed completely, much like the “star field” test at the top of the article.
We perceive patterns in randomness, and it extends beyond casual situations like basketball punditry, plaguing us even when we’re trying to be intentionally, objectively analytical.
Take the “interesting statistic” given by the baseball announcers in the first example above. Sure the last 5 of 7 batters were retired, but the act of picking the number 7 implies that number 8 got on base, and so did the one before the sample window. So it’s at least “5 of 9,” not “5 of 7,” but that doesn’t sound as impressive, even though it’s the same data.
Unlike the basketball example, the baseball announcer’s error runs deeper, and following that thread will bring us to our own marketing data and the heart of the fallacy.
Seeking combinations
Baseball statisticians record a dizzying smorgasbord of statistics that announcers eagerly regurgitate. Maybe it’s because baseballers are a little OCD (evidenced by pre-bat and pre-pitch rituals) or maybe it’s because they need something to say to soak up the time between pitches, but in any case the result is a mountain of data.
Announcers exploit that data for the most esoteric of observations:
“You know, Rodriguez is 7 for 8 against left-handed pitchers in asymmetric ballparks when the tide is going out during El Niño.”
This is the epitome of Pattern-Seeking—combing through a mountain of data until you find a pattern.
Some statistician examined millions of combinations of player data and external factors until he happened across a combination which included a “7 of the last 8,” which sure sounds impressive and relevant. Then he proudly delivered the result as if it were insight.
So what’s wrong with stumbling across curious observations? Isn’t that how you make unexpected discoveries?
No, it’s how to convince yourself you’ve made a discovery when in fact you’re looking at pure randomness. Let’s see why.
Even a fair coin appears unfair if you’re Pattern-Seeking
The fallacy is clearer when you look at an extreme yet accurate analogy.
I’m running an experiment to test whether a certain coin is biased. During one “trial” I’ll flip the coin 10 times and count how often it comes up heads. 5 heads out of 10 would suggest a fair coin; so would 6 or even 7, due to typical random variation.
What if I get 10 heads in a row? Well a fair coin could exhibit that behavior, but it would be rare—a 1 in 1024 event. So if my experiment consists of just one trial and I get 10 heads, the coin is suspicious.
But suppose I did a “10-flip trial” one thousand times. A fair coin should still come up heads 3-7 times in each trial, but every once in a while it will come up 9 or 10 times. Those events are rare, but I’m flipping so much that rare events will naturally occur. In fact, in 1000 trials there’s a 62% chance that I’ll see 10 heads at least once.
This is the crux of the fallacy. When an experiment produces a result that is highly unlikely to have happened by chance, you conclude that something systematic is at work. But when you’re “seeking interesting results” instead of performing an experiment, highly unlikely events will necessarily happen, yet still you conclude something systematic is at work.
Indeed, this is one reason why scaling a business is so hard: Scale makes rare things common.
Bringing it home to marketing and sales data
Let’s apply the general lesson of the coin-flipping experiment to Google Analytics. There’s a hundred ways to slice and dice data, so that’s what you do. If you compare enough variables enough ways, you’ll find some correlations:
“Oh look, when we use landing page variation C along with AdWord text F, our conversion rate is really high on Monday mornings.”
Except you sound just like the baseball announcer, tumbling combinations of factors until something “significant” falls out.
Except you’re running 1000 coin-flip trials, looking only at the trial where it came up all heads and declaring the coin “biased.”
Except you’re seeing streaks, hoping that this extra-high conversion rate is evidence of a systematic, controllable force.
The solution: Form a theory, test the theory
You can use apparent patterns to form a theory; that’s good.
The most exciting phrase in science isn’t “eureka,” but rather, “that’s funny.
—Isaac Asimov
But then you have to test it, so that it’s science, not baseball announcing.
So:
- Instead of using a thesaurus to generate 10 ad variants, decide what pain-points or language you think will grab potential customers and test that theory specifically.
- Instead of rooting around Google Analytics hoping to find a combination of factors with a good conversion rate, decide beforehand which conversion rates are important for which cohorts, then measure and track those only.
- Instead of asking customers leading questions or collecting scattered thoughts, form hypotheses and intentionally test them.
- Instead of blindly following the startup founder who dramatically succeeded (the 1-in-1000 coin flip?), gather advice and observations that align with your style and goals.
And then never stop testing your theories, because you never know when the environment changes, or you change, or you find something even better.
There are two possible outcomes: if the result confirms the hypothesis, then you’ve made a measurement. If the result is contrary to the hypothesis, then you’ve made a discovery.
—Enrico Fermi
https://longform.asmartbear.com/pattern-seeking-fallacy/
© 2007-2024 Jason Cohen
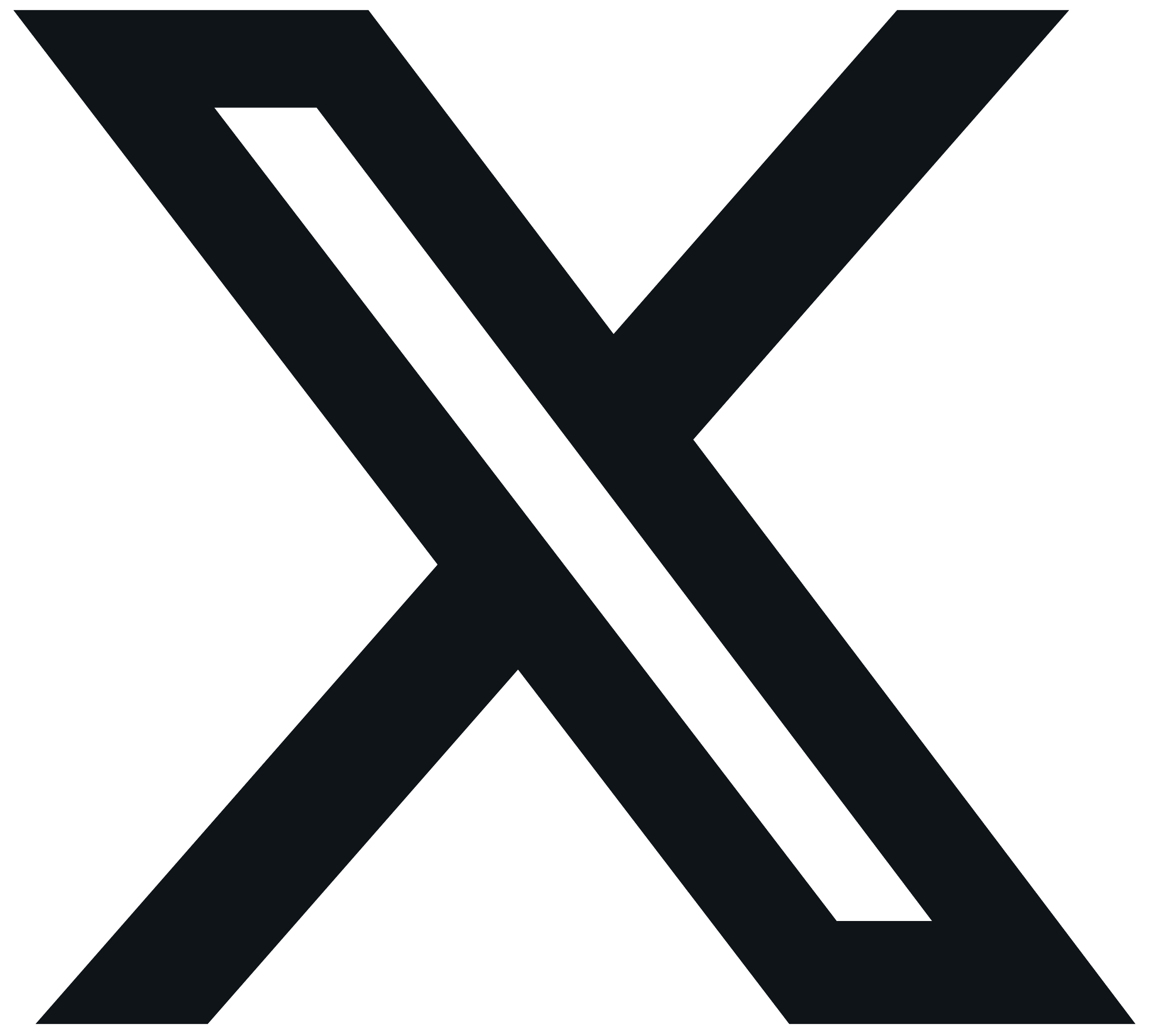